Let’s define “clinical AI” for mental healthcare
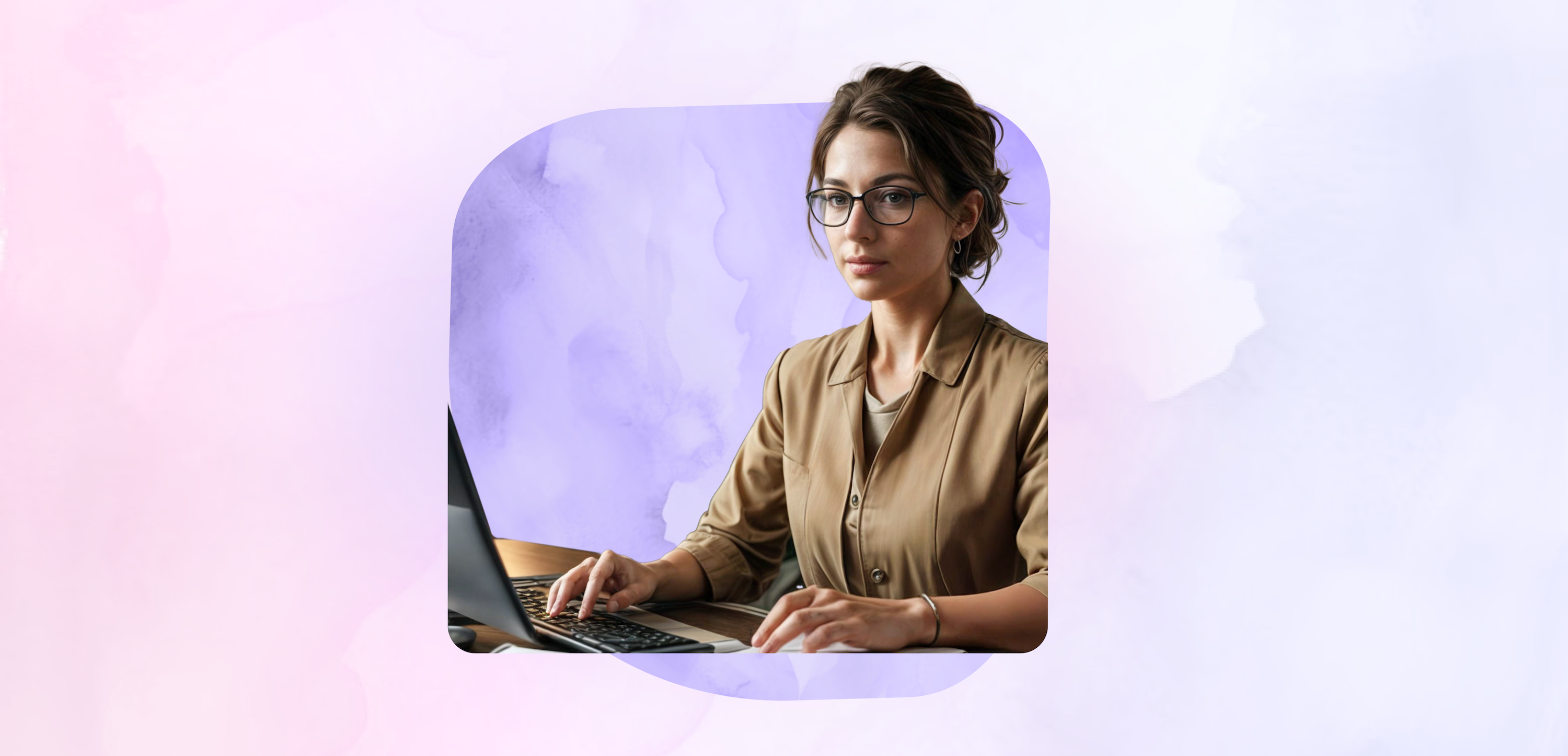
If you’ve spent much time around Limbic, you’ll have heard or read the term “clinical AI.” Initially, we relied on this phrase to differentiate the patient-facing clinical AI tools that are central to our mission from the administrative AI products that have become commonplace in mental healthcare (think: notetakers, billing tools, etc.).
Both categories have their place. But by the very nature of what Limbic’s core AI tools offer—patient assessments, diagnostic decision support for therapists, continuous patient support between therapy sessions—clinical accuracy and patient safety have to guide everything we do. We are a clinical AI company, full stop.
This is how we define that work.
What Is Clinical AI?
In broadest terms, clinical AI is artificial intelligence deployed in clinical settings to support the direct delivery of care and improve patient outcomes. Unlike AI used for administrative or operational purposes—like note-taking and billing—clinical AI augments clinical decision-making, treatment planning, and patient care. This includes applications such as:
- Diagnostics: AI models that assist clinicians in diagnosing diseases and conditions by analyzing imaging data, symptoms, or behavioral patterns.
- Treatment recommendations: Systems that analyze clinical data and offer personalized treatment plans.
- Therapeutic interventions: AI-powered apps and assistants that help deliver those plans directly to patients and track their progress.
- Patient monitoring: AI-driven systems that track patients' vitals, monitor changes, and/or predict potential risks and complications.
- Predictive analytics: Predicting the likelihood of specific events or outcomes, based on patient history and large datasets.
- Usually patient-facing, as well as provider-facing. Since clinical AI should have a material effect on patient outcomes, it usually has some degree of patient interaction. “Patient” is key here. Clinical AI is designed for healthcare and clinical settings, rather than general wellness apps.
We add additional layers to that. For us, “clinical AI” should also indicate:
- Robust and ongoing scientific research: Clinical AI is underpinned by rigorous clinical evidence. Look for published, peer-reviewed research in respected journals, along with a pipeline of ongoing research and submissions. These studies should have very large sample sizes and be conducted in clinical settings.
- Rigorous safety testing: Before launching even a new product feature—let alone an entire product—we evaluate safety and reliability across tens of thousands of interactions.
- Third-party validation: Companies claiming “clinical AI” should meet the standards set by outside organizations whose priorities have nothing to do with those companies’ commercial interests and everything to do with data security, user privacy, patient safety, and clinical precision.
- Regulatory compliance: While “clinical AI” isn’t defined and regulated by any government agencies (like, say, “organic” and the U.S. Department of Agriculture), companies using this term should adhere to HIPAA, GDPR, and other relevant regulatory frameworks. When you move out of wellness and into healthcare, you have to meet higher standards.
How is clinical AI used in mental healthcare?
- Diagnostic decision support. Where AI note takers work behind the scenes as an administrative assistant, clinical AI operates as a true clinical companion in frontline care. It’s not a diary but, rather, a diagnostic support tool.
- Patient assessments and conversational support. Clinical AI is often, though not always, employed conversationally, in patient-facing settings. This requires additional and complex guardrails to ensure patient safety and clinical precision.
- Treatment planning. Because of the massive datasets it employs, its robust probabilistic models, and the guardrails built around its recommendations, clinical AI can support not just assessments but ongoing treatment planning and delivery.
- Clinical efficiency. By speeding up assessments and diagnoses, these tools hand clinical time back to mental health services, which can be used to reduce the burden on care providers.
- Patient outcomes. The diagnostic precision that true clinical AI tools can deliver has a compounding effect on patient outcomes. It leads to better therapist matching, fewer re-assessments, and more effective treatment planning, meaning more appropriate care right from the start. This reduces dropouts and no-shows, which, in turn, can lead to better and faster outcomes for patients.
- Asynchronous care. The understandable focus on conversation as the core of the therapy experience can limit access, due to both working hours and the simple fact that there aren’t enough therapists to meet demand. Clinical AI can immediately alleviate both strains on the system.
- Personalization. While any large-language model AI can respond contextually to user inputs, clinical AI tools have proprietary, purpose-built algorithms and models in place to ensure that responses are clinically appropriate, evidence-based, and aligned with treatment plans.
Where is clinical AI for mental healthcare headed?
Humanity doesn’t merely adopt digital technologies; it does so at an accelerating rate. It took more than 20 years after the introduction of consumer-grade personal computers for that technology to reach a 50% adoption rate in the US. It was just under a decade for the World Wide Web. For generative AI: Two years.

The takeaway: Consumers are already relying more and more on AI in their daily lives, including in healthcare. And clinical AI companies are already improving precision and outcomes in healthcare settings.
As long as companies operating in this space prioritize patient safety and clinical precision above all else, clinical AI can exponentially expand access to the highest quality mental healthcare and reduce the burden on providers—all while improving patient outcomes.